Northwestern and CIERA understand the tremendous scientific opportunities artificial intelligence (AI) brings. AI science is a critical research area at CIERA, especially in big data, transient science, image processing, and numerical simulations. CIERA members are active in AI research and have committed time and resources to maximize the scientific return with AI.
AI Science at CIERA
Emma Alexander’s research group is motivated by biology to create novel, low-level, physics-based computer vision tools. A new understanding of animals’ brains and behavior can inspire new technologies, particularly in the imaging and image processing domains. The group contributes to the next generation of cameras and displays by reverse-engineering principles of natural vision.
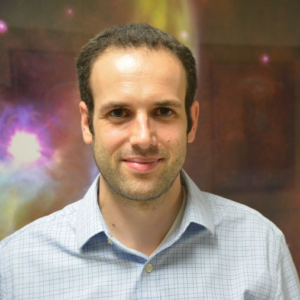
Claude-André Faucher-Giguère’s research group develops cosmological simulations that model the formation of galaxies from the Big Bang to the present time. The group is interested in various aspects of how machine learning can advance or accelerate science based on numerical simulations. One example is the development of emulators (or surrogate models) for predictions from large cosmological simulations for upcoming galaxy surveys (including with the Rubin and Roman telescopes) and cosmic microwave background experiments. This group collaborates closely with scientists from the Cosmological Physics and Advanced Computing group at Argonne National Laboratory. Faucher-Giguère is also interested in how machine learning can potentially accelerate multi-scale simulations, such as by using machine learning models to capture unresolved physics.
Vicky Kalogera’s research group explores massive binary star systems’ lives, properties, and fates through theoretical and empirical approaches. To overcome the computational demands of simulating stellar structure and intricate binary interactions, the group employs machine learning tools within the POSYDON framework to efficiently emulate simulation outcomes. Additionally, the group uses machine-learning techniques to characterize gravitational waves generated when black holes or neutron stars from these systems collide. They also contribute to the Gravity Spy public science project, facilitating collaboration among machine-learning experts, gravitational-wave scientists, and volunteers from the public to classify and comprehend noise sources in gravitational-wave detectors.
Aggelos Katsaggelos’ research group conducts cutting-edge research to address the current challenges and applications of image and video processing. His group primarily focuses on machine learning and other data science techniques to solve these problems, and much of our research has been published in top journals and conferences.
Adam Miller’s research group works at the intersection of wide-field time-domain astronomical surveys and data science. To this end, machine learning models are constructed to classify billions of sources in astronomical imaging data to prioritize which sources will prove the most valuable for spectroscopic follow-up observations, which require more resources than just obtaining an image. The group is currently developing multi-input convolutional neural networks to predict the maximum brightness of newly discovered stellar explosions and variables in nightly images from the Zwicky Transient Facility.
Tjitske Starkenburg studies galaxy evolution and dynamics and applies various data science and machine learning techniques to untangle the many physical processes at play. Making mock observations from simulated galaxies and their dynamical structures combined with using likelihood-free inference techniques can highlight underlying cross-dependencies and test physical models. Starkenburg is also interested in developing differentiable models, in using machine learning to accelerate and emulate simulations, and to improve detection and characterization of the faintest galaxy structures.
Jason Wang’s research group works on discerning and isolating the faint light of exoplanets orbiting their bright host stars to image these exoplanets directly. In addition to advancing novel instrumentation and technology, the group uses blind signal separation and other data-driven image processing techniques to remove the star’s glare, which can be a million times brighter than the planets orbiting it. The group is currently developing image processing techniques for the next generation of exoplanet imaging instruments, including deep learning for image reconstruction to aid in removing the star’s bright glare.
Training the Next Generation of AI Scientists
CIERA has integrated AI science into the training and research experiences of the next generation of astronomers, including undergraduate students, graduate students, and postdocs.
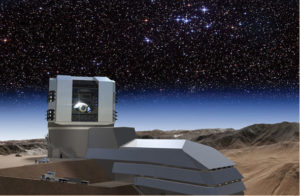
LSSTC Data Science Fellowship Program
CIERA’s data science expertise is leveraged to advance the Legacy Survey of Space and Time (LSST) science and student training through the LSSTC Data Science Fellowship Program. Prof. Adam Miller is the Program Director for the LSSTC Data Science Fellowship Program, a training fellowship for graduate students in astronomy and related fields that aims to teach cutting-edge methods in data science in advance of the LSST.
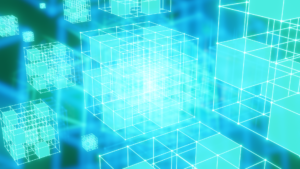
Integrated Data Science (Certificate)
Initially created at CIERA, the Integrated Data Science Certificate bridges several disciplines and pushes research frontiers: from the methods disciplines of computer science, electrical engineering, applied math, and statistics to domain disciplines across science and engineering. The certificate in Integrated Data Science aims to organize and recognize students, integrate curriculum in methods and domain disciplines, and offer students the wide range of education they need to succeed as data scientists both inside and outside academia.